Empathetic Dialogue Generation
A humanized dialogue system is expected to generate empathetic replies, which should be sensitive to the users’ expressed emotion. The task of empathetic dialogue generation is proposed to address this problem. The essential challenges lie in (1) accurately capturing the nuances of human emotion, (2) modelling complex emotional dependencies between conversation partners, and (3) considering the potential of user feedback, which are overlooked by the majority of existing work. In response to this problem, we propose two empathetic dialogue generation frameworks in two different directions.
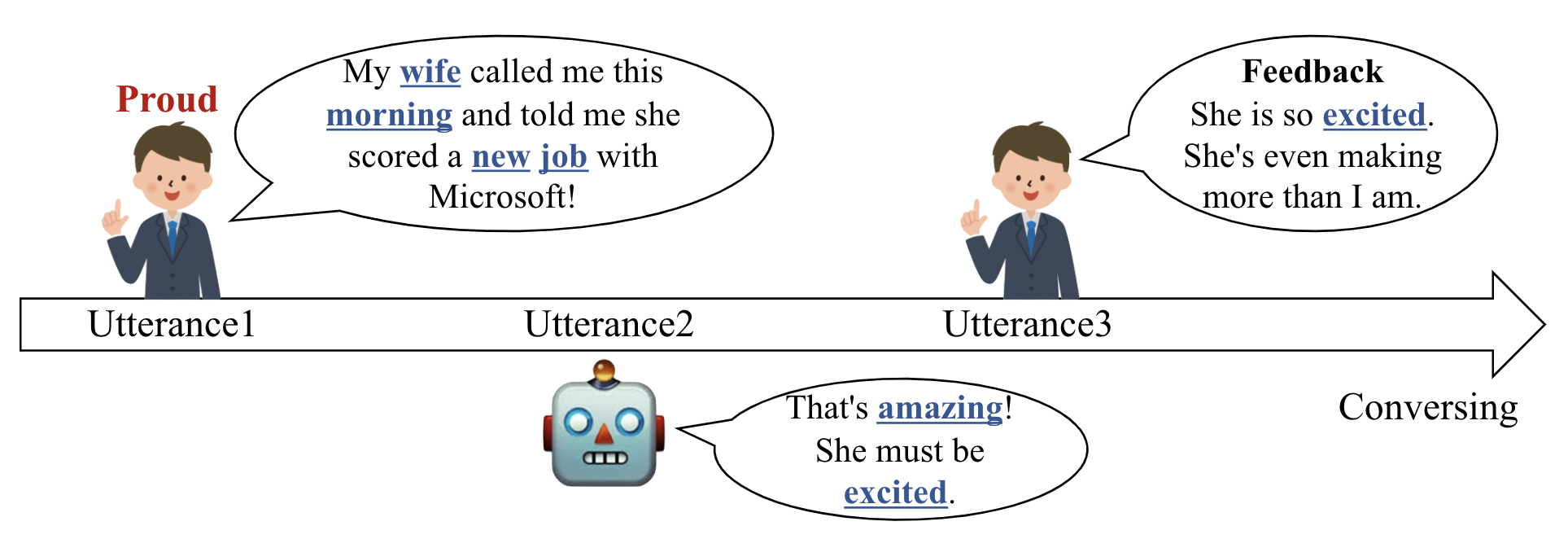
As shown in Figure 1, we list an example from the benchmark dataset EmpatheticDialogues (Rashkin et al., 2019). Notably, emotional words in the three serial utterances between interlocutors have nuanced emotional connections, i.e., “new, job” in utterance 1, “amazing, excited” in utterance 2, and “excited” in utterance 3. Without considering fine-grained emotional words, the responses generated by existing methods are trivialand uninformed, even though they expressed the appropriate emotions. Therefore, explicitly modellingthe fine-grained emotional factor is necessary.
We propose a multi-resolution adversarial model – EmpDG, to generate more empathetic responses, which is accepted by COLING 2020.
EmpDG exploits both the coarse-grained dialogue-level and fine-grained token-level emotions, the latter of which helps to better capture the nuances of user emotion. In addition, we introduce an interactive adversarial learning framework which exploits the user feedback, to identify whether the generated responses evoke emotion perceptivity in dialogues. Experimental results show that the proposed approach significantly outperforms the state-of-the-art baselines in both content quality and emotion perceptivity.
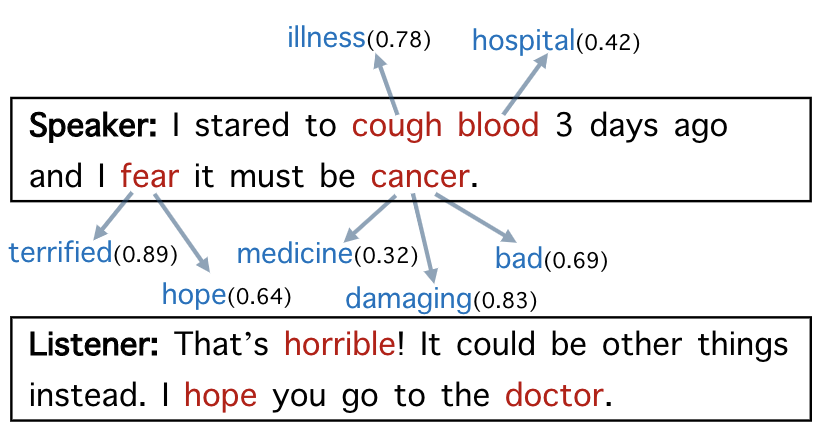
Lacking external knowledge makes it difficult to perceive implicit emotionsfrom limited dialogue history. To address the above challenges,we propose to leverage multi-type knowledge, i.e, the commonsense knowledge and emotional lexicon, to explicitly understand and express emotions in empathetic dialogue generation.
We propose a multi-type knowledge aware empathetic dialogue generation framework, to explicitly understand and express emotions in empathetic dialogue generation, which is submitted to AAAI 2021.
We first enrich the dialogue history by jointly interactingwith two-type knowledge and construct an emotional contextgraph. Then we introduce a multi-type knowledge-aware context encoder to learn emotional context representations anddistill emotional signals, which are the prerequisites to predicate emotions expressed in responses. Finally, we proposean emotional cross-attention mechanism to exploit the emotional dependencies between the emotional context graph andthe target empathetic response. The proposed framework makes it easier to accurately perceive and appropriately express implicit emotions.